Unmasking the Invisible: How Machine Learning is Revolutionizing Scientific Anomaly Detection
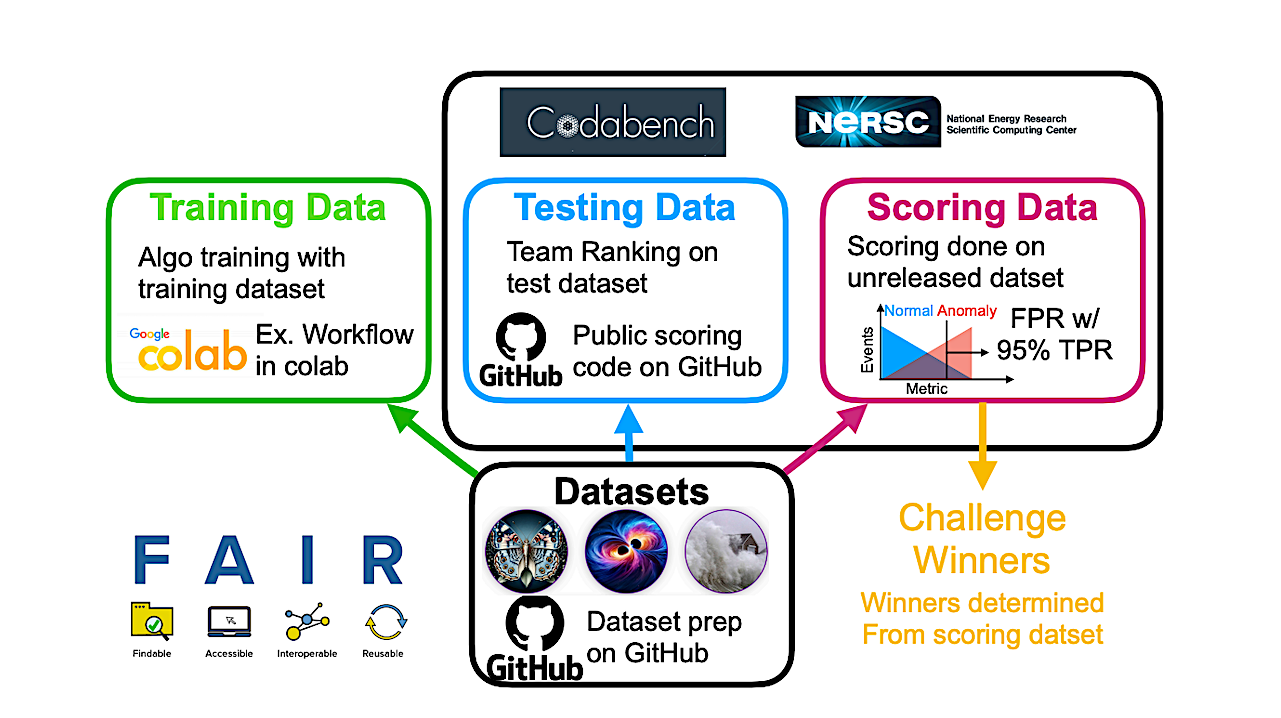
The world of scientific discovery is a thrilling landscape of unexpected revelations, where breakthrough moments often emerge from the most surprising places. Scientists frequently make groundbreaking advances by uncovering patterns or observing phenomena that challenge existing scientific understanding. These remarkable moments occur when researchers step beyond the boundaries of established theories, revealing hidden connections and insights that were previously invisible.
Imagine a researcher peering through a microscope or analyzing complex data, suddenly recognizing something that defies current scientific knowledge. Such discoveries are not just random accidents, but the result of keen observation, curiosity, and a willingness to question established norms. Whether in physics, biology, astronomy, or any other scientific field, these unexpected findings can revolutionize our understanding of the universe and how it operates.
The beauty of scientific exploration lies in its ability to constantly reshape our comprehension of reality. When a pattern or object emerges that cannot be explained by existing scientific rules, it opens up new avenues of research, sparks innovative thinking, and pushes the boundaries of human knowledge. These moments remind us that science is not a fixed set of immutable laws, but a dynamic, ever-evolving process of discovery and understanding.