Fortress Finance: How Manufacturers Are Shielding Sensitive Data from Cyber Threats
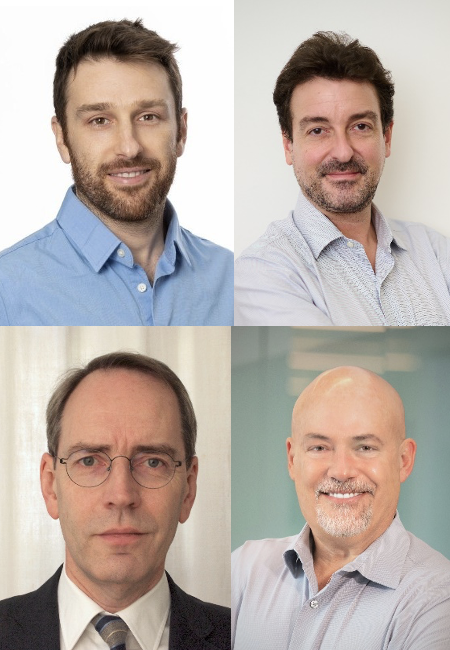
In the rapidly evolving landscape of technology, data sharing has become increasingly complex, particularly with the emergence of advanced artificial intelligence and sophisticated multi-die semiconductor assemblies. These cutting-edge technologies are pushing the boundaries of traditional data exchange methods, presenting unprecedented challenges for engineers and researchers.
Multi-die assemblies, which combine multiple semiconductor chips into a single integrated package, introduce intricate data transfer and synchronization challenges. The complexity stems from the need to manage diverse chip architectures, communication protocols, and performance requirements. Meanwhile, artificial intelligence systems demand unprecedented levels of data precision, bandwidth, and real-time processing capabilities.
The intersection of AI and multi-die technologies creates a perfect storm of data sharing obstacles. Engineers must now navigate intricate technical landscapes, balancing performance optimization with seamless data integration. Innovative approaches like advanced interconnect technologies, heterogeneous computing architectures, and intelligent data routing mechanisms are emerging as potential solutions.
As the technological frontier continues to expand, organizations and researchers must develop more sophisticated strategies for data sharing. Collaboration, standardization, and continuous technological innovation will be crucial in overcoming these complex challenges and unlocking the full potential of AI and multi-die semiconductor technologies.