Digital Twin Revolution: Critical Manufacturing and Twinzo Unveil Cutting-Edge Visualization Tech
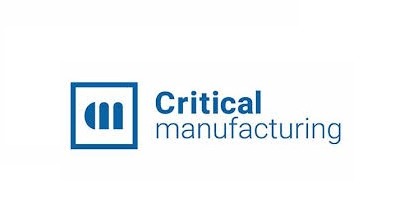
Pioneering Digital Twin Technology: A Strategic Manufacturing Partnership
In a groundbreaking collaboration, industry leaders are joining forces to accelerate the integration of digital twin technology within cutting-edge smart manufacturing environments. This innovative partnership represents a significant leap forward in transforming traditional manufacturing processes through advanced digital simulation and modeling techniques.
Digital twin technology enables manufacturers to create virtual replicas of physical systems, allowing for unprecedented insights, predictive maintenance, and optimization of production workflows. By leveraging real-time data and sophisticated simulation capabilities, companies can now simulate complex manufacturing scenarios, identify potential inefficiencies, and make data-driven decisions with remarkable precision.
The strategic alliance aims to drive technological innovation, enhance operational efficiency, and provide manufacturers with powerful tools to remain competitive in an increasingly digital industrial landscape. Through this partnership, organizations can unlock new levels of performance, reduce downtime, and streamline their manufacturing processes like never before.